AI tools are revolutionizing user research by offering innovative ways to collect, analyze, and act on data. Whether you’re a seasoned professional or just starting to explore the potential of AI, this guide offers a mix of advanced strategies and accessible tips to improve your user research practice.
Part 1: Advanced AI Strategies for User Research
AI is changing the way user research is conducted, making it faster, smarter, and more insightful. While premium tools like Dovetail, Hotjar, and Maze are common in many workflows, here’s how to leverage them and other cutting-edge strategies for maximum impact, regardless of your resources.
1. Emotional journey mapping
What it means: Beyond simple sentiment analysis, advanced AI can map the nuanced emotional journey of users as they interact with a product or service.
Example: Affectiva can analyze micro-expressions from video recordings of user interviews or usability tests, identifying emotions like frustration, joy, or confusion. Similarly, Hotjar by Contentsquare uses heatmaps and session recordings to reveal behavioral patterns, showing where users hesitate, click repeatedly, or drop off.
How to use: Incorporate these insights into your UX design process by addressing friction points or enhancing features that evoke positive emotions.
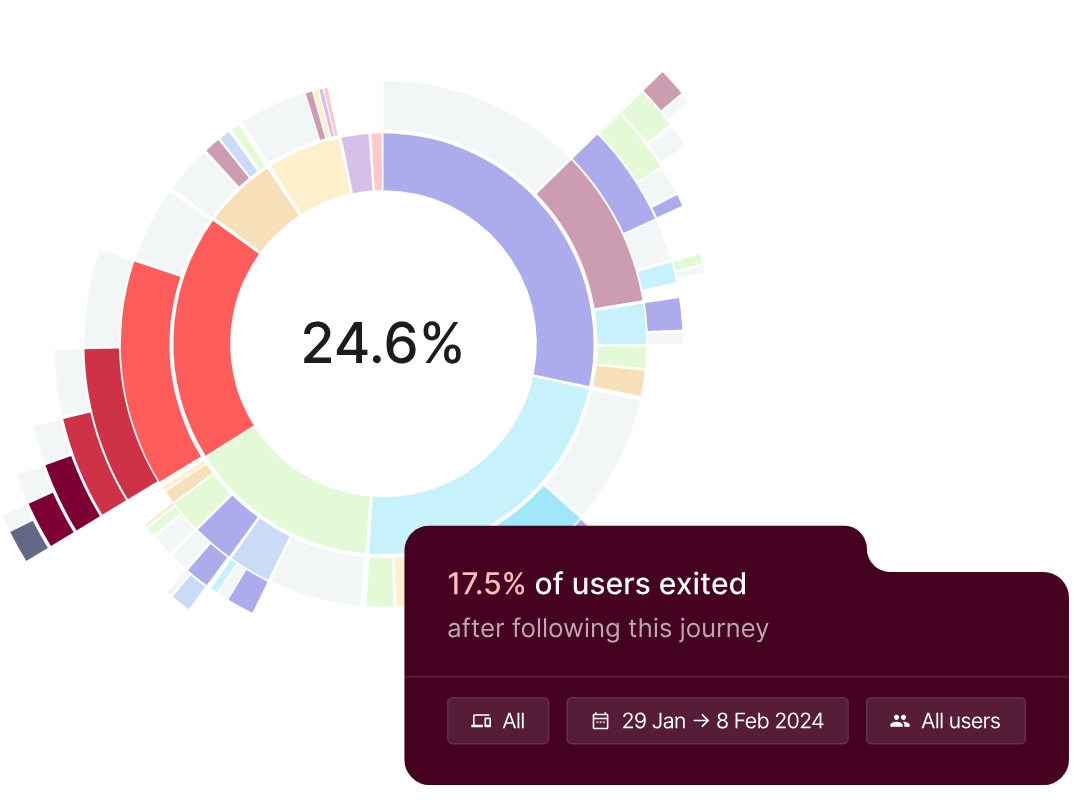
2. Predictive behavioral modeling
What it means: Instead of just analyzing past behavior, AI can predict future user actions, preferences, and potential areas of friction.
Example: By combining AI tools like Mixpanel (behavioral analytics) and reinforcement learning models, you can simulate how users might interact with a new feature before rolling it out. This is especially powerful for optimizing conversion paths or reducing churn.
How to use: Run predictive models during the prototyping phase to identify and mitigate potential usability roadblocks.
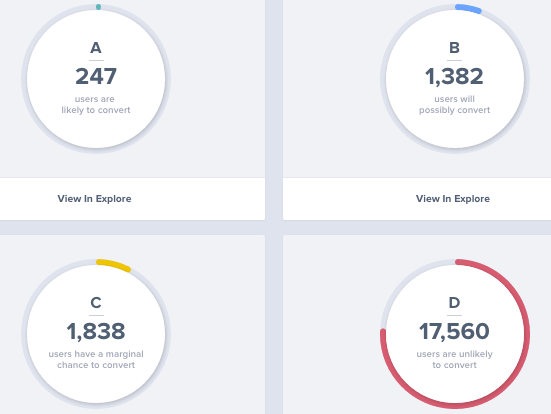
3. Data synthesis across research sources
What it means: AI tools can aggregate and synthesize data from disparate sources-user interviews, surveys, usability tests, and analytics platforms-into a unified view.
Example: Platforms like EnjoyHQ can integrate qualitative data (e.g., interview transcripts) with quantitative metrics (e.g., website heat maps or click-through rates). Using GPT-powered summaries, researchers can quickly generate cross-source insights that would take hours to generate manually.
How to use: Use data synthesis to create comprehensive research reports that combine storytelling with hard metrics for stakeholder presentations.
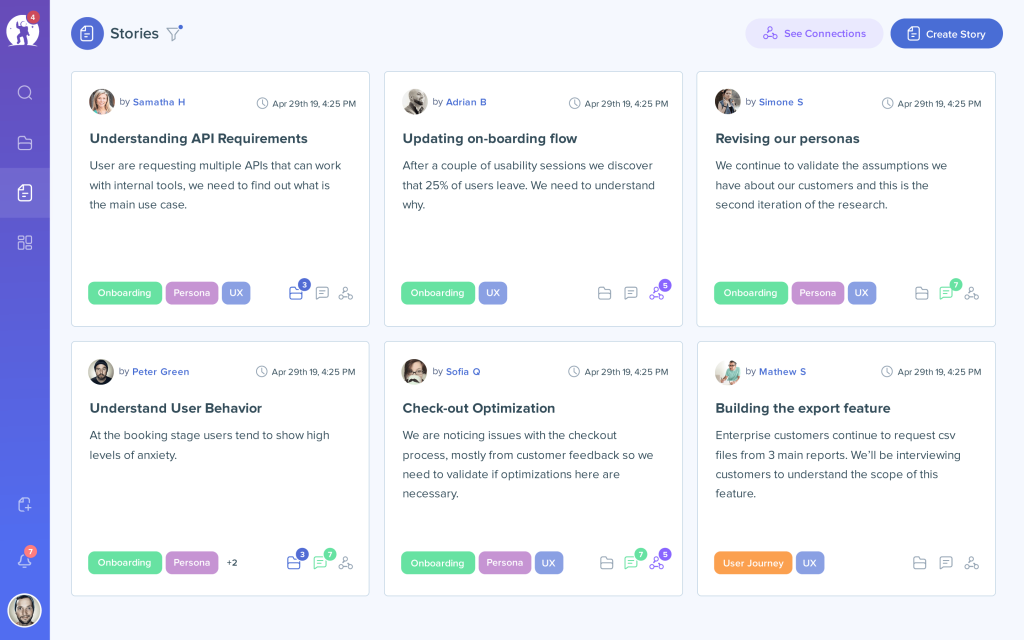
4. Multilingual user research at scale
What it means: Conducting research across regions and languages can be time-consuming. AI can streamline this process by translating and analyzing responses without losing cultural nuances.
Example: Tools like DeepL for translation paired with NLP models can analyze sentiment and themes in multiple languages, making global user research both faster and more accurate.
How to use: Use multilingual AI tools when scaling products for international markets to ensure the cultural relevance of your findings.
5. Competitive benchmarking with AI
What it means: AI enables dynamic, automated competitor analysis that can track changes over time in user flows, pricing strategies, or feature updates.
Example: Instead of static competitor audits, use AI-powered web scraping tools like Crayon or SEMrush combined with GPT models to create ongoing dashboards that monitor competitors’ UX, marketing, and design changes.
How to apply: Use these insights to maintain a competitive edge by iterating based on proven market trends.
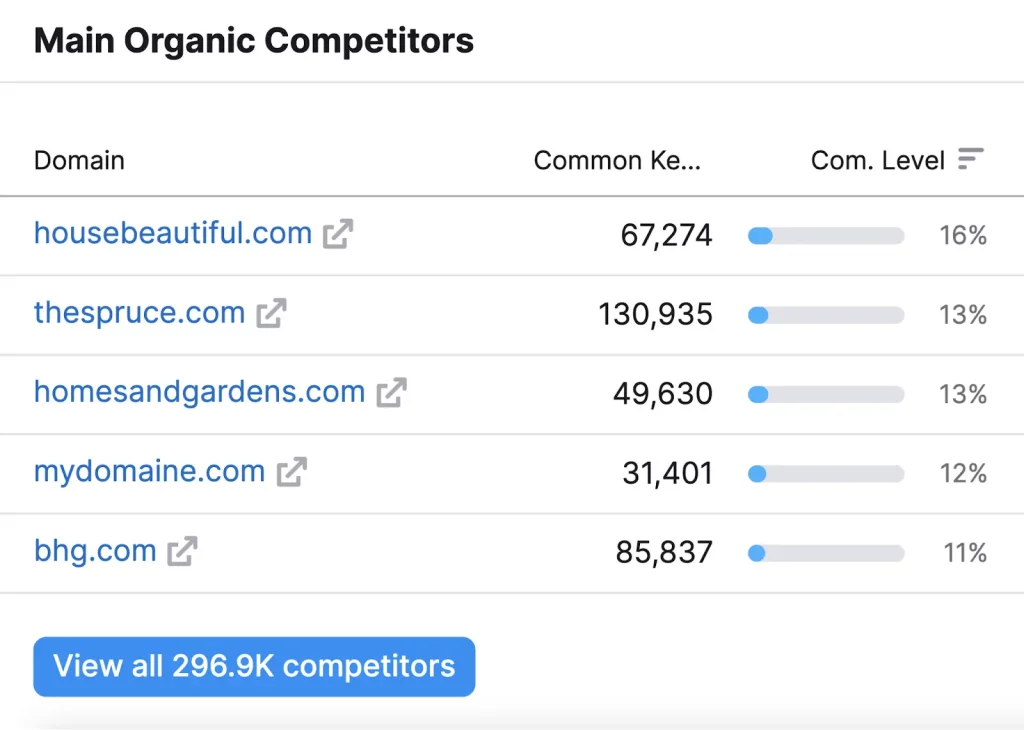
Part 2: Practical Cheat Sheet on Microsoft Copilot for Strategic User Research
Copilot can be a game changer for product designers by providing a quick way to generate and refine user research components. Here’s how to use it effectively, centered around the scenario of designing a budgeting app.
1. Generate assumption maps
Prompt Example:
“We’re designing a budgeting app for individual users. Draft ten assumptions about their needs, motivations, and challenges when managing personal finances.”
Use Case: Develop hypotheses about user needs, such as users prioritizing ease of tracking expenses or preferring automated saving recommendations.
2. Draft research plans
Prompt Example:
“Based on these assumptions: [list assumptions], create a user research plan. Include the methods (e.g., interviews, surveys), sample sizes, and specific objectives for each method.”
Use Case: Create a structured plan to validate user assumptions efficiently, aligned with project goals.
3. Write interview questions
Prompt Example:
“We are conducting interviews to validate user requirements for our budgeting application. Write a complete interview guide, including a briefing, warm-up questions, and key questions that focus on financial habits and challenges.“
Use Case: Save time on interview prep while ensuring a thorough exploration of user pain points.
4. Create testing scenarios
Prompt Example:
“Our team has designed a prototype for the budgeting app. Create a usability test scenario, including specific tasks such as adding an expense, setting a savings goal, and tracking progress.”
Use Case: Design targeted usability tests to uncover friction points in core workflows.
5. Develop personas
Prompt Example:
“We anticipate three user types for our budgeting app: 1. Savers who focus on long-term goals, 2. Spenders looking to reduce monthly expenses, and 3. Young professionals seeking financial literacy. Create detailed personas for these groups.”
Use Case: Quickly generate personas with motivations, goals, and frustrations to inform design decisions.
6. Summarize and analyze qualitative data
Prompt Example:
“We conducted interviews about the budgeting app. Here’s the transcript: [insert]. Summarize key themes and highlight the most common user pain points.”
Use Case: Accelerate data synthesis with a focus on actionable insights.
7. Run Sentiment Analysis
Prompt Example:
“Analyze this interview transcript from a budgeting app user for sentiment. Identify positive, negative, and neutral feedback.”
Use Case: Extract sentiment trends to inform design refinements.
8. Generate Reports
Prompt Example:
“Draft a PowerPoint outline for presenting user research findings on the budgeting app, including sections for assumptions, methods, insights, and design recommendations.”
Use Case: Streamline report creation for stakeholder presentations.
9. Conduct Competitor Analysis
Prompt Example:
“Analyze the features and user flows of competitor budgeting apps like [Competitor A, B, C]. Highlight similarities, differences, and potential areas where our app can differentiate.”
Use Case: Identify opportunities for competitive advantage through a detailed feature and UX comparison.
While these prompts can also be used with ChatGPT, a widely used AI tool, our UX community uses Microsoft Copilot for an added layer of security. Copilot operates in a protected environment which ensures that sensitive data and insights remain secure throughout the research process. This emphasis on data security is why we recommend using Microsoft Copilot when applying these techniques in a professional setting.
Final Thoughts
AI, from advanced tools to accessible platforms like Copilot, empowers product designers to uncover user insights and make data-driven decisions. Whether you’re using predictive analytics or crafting interview questions, these strategies enable teams to create user-centered designs that truly resonate.
Interested to get more professional insights like this article? Good news, our Product Design community shares them monthly in our Product Design Newsletter, to which you can subscribe here.